3D Printing Monitoring System Based on Supervised Machine Learning
March 31, 2023 | Vol.9, No.3 | PP. 1-10 | PDF
AUTHORS:
Ho Jong Song, Department of Industrial Engineering, Kumoh National Institute of Technology, Republic of Korea
Tae Sung Kim, Department of Industrial Engineering, Kumoh National Institute of Technology, Republic of Korea
Goo Sang Jung, Conception Co., Ltd., Republic of Korea
Jin Ung Jeon, Conception Co., Ltd., Republic of Korea
KEYWORDS:
Tensile Strength, Overlap Rate, Scan Speed, Laser Power
Abstract
One of the additive manufacturing processes that is quickly evolving is metal 3D printing. Selective laser melting (SLM) is one of the popular methods for metal 3D printing. Although it has many advantages and capabilities, there are still unsolved problems like process monitoring and printing reliability. Printing high-quality robust products require appropriate parameter settings and real-time monitoring. In order to satisfy the requirement, we propose a new monitoring system based on multiple sensors that can measure the index of different quality affecting parameters of SLM 3D printing. The system serves to improve printing quality; it involves supervised machine learning to predict the expected tensile strength of the printed product. We trained the machine learning model on our new “tensile strength” dataset which includes multiple sensing data and indexes of tensile strength. While collecting data we printed products that have a tensile strength between 449 and 506 MPa. A number of SLM 3D printing tests are carried out to show the viability of the proposed approach. After testing the tensile strength of the printed product, test results were compared to the results of the tensile strength predicting model. According to experiments, the monitoring system showed satisfactory results predicting expected tensile strength. The highest accuracy has been achieved with Multiple Linear Regression, recording 97%. The monitoring system helps not only to predict the tensile strength of the printing product but also to find optimal parameter settings of the SLM printer.
APJCRI
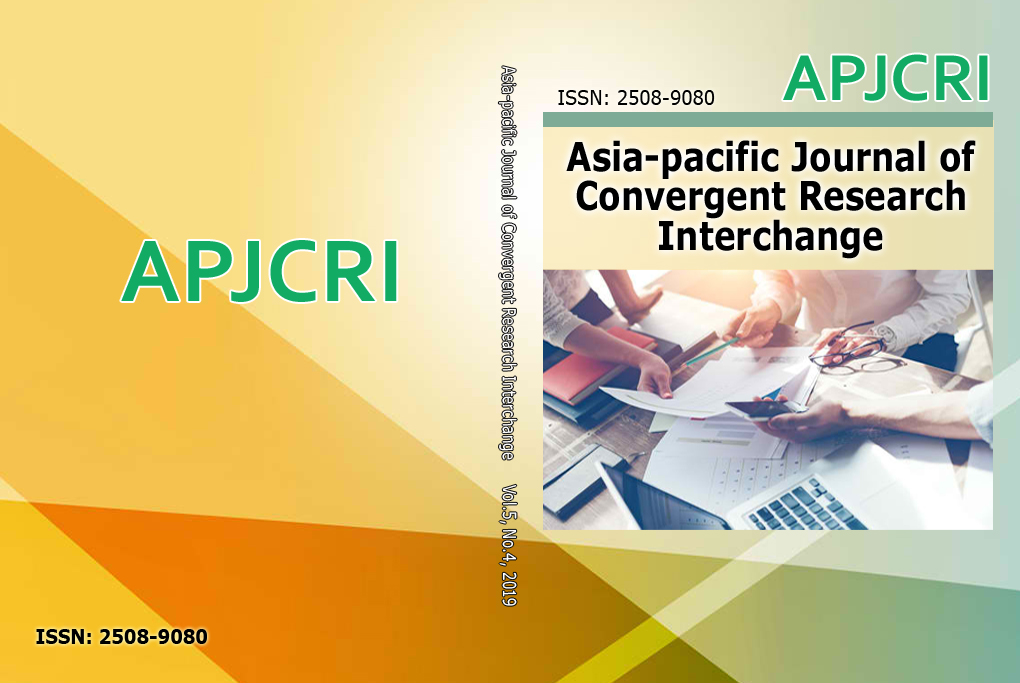
The purpose of this journal is to share and exchange the results of recent research by Asia-Pacific national researchers, and the purpose of the project is to publish the results of convergence research with the humanities and social sciences, including advanced research in specific fields.